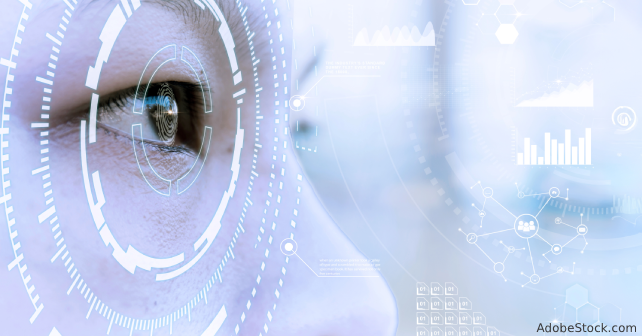
I am preparing to review another systematic review and meta-analysis (SRMA). Although it is enjoyable to critically appraise these publications, I need to verify whether the authors followed the Preferred Reporting Items for Systematic reviews and Meta-Analyses (PRISMA) guidelines. There has been a lot of enthusiasm for open-source large language models (LLMs), like ChatGPT 3.5 from Open AI, and I wonder if artificial intelligence (AI) could do this task quickly and accurately.
Explore This Issue
ACEP Now: Vol 44 – No 01 – January 2025Background
LLMs such as ChatGPT and Claude have shown remarkable potential in automating and improving various aspects of medical research. One interesting area is their ability to assist in critical appraisal, one of the core aspects of evidence-based medicine. Critical appraisal involves evaluating the quality, validity, and applicability of studies using structured tools like PRISMA, AMSTAR (A MeaSurement Tool to Assess systematic Reviews), and PRECIS (PRagmatic Explanatory Continuum Indicator Summary)-2.
Confirming adherence to quality checklist guidelines often requires significant expertise and time. However, LLMs have evolved to interpret and analyze complex textual data and therefore represent a unique opportunity to enhance the efficiency of these appraisals. Research into the accuracy of LLMs for these tasks is still in its early stages.
Clinical Question
Can LLMs accurately assess critical appraisal tools when evaluating systematic reviews and randomized controlled trials (RCTs)?
Reference
Woelfle T, Hirt J, Janiaud P, et al. Benchmarking human–AI collaboration for common evidence appraisal tools. J Clin Epidemiol. 2024;175:111533.
Population: Systematic reviews and RCTs that were evaluated by critical appraisal tools (PRISMA, AMSTAR, and PRECIS-2).
Intervention: Five different LLMs—Claude 3 Opus, Claude 2, ChatGPT 4, ChatGPT 3.5, Mixtral-8x22B—assessing these studies.
Comparison: Comparisons were made against individual human raters’ human consensus ratings and human–AI collaboration.
Outcome: Accuracy and identification of potential areas for improving efficiency via human–AI collaboration.
Authors’ Conclusions
“Current LLMs alone appraised evidence worse than humans. Human–AI collaboration may reduce workload for the second human rater for the assessment of reporting (PRISMA) and methodological rigor (AMSTAR), but not for complex tasks such as PRECIS-2.”
Results
The authors assessed 113 SRMAs and 56 RCTs. Humans had the highest accuracy for all three assessment tools. Of the LLMs, Claude 3 Opus consistently performed the best across PRISMA and AMSTAR, indicating that it may be the most reliable LLM for these tasks.
The older, smaller model, ChatGPT 3.5, performed better than newer LLMs like ChatGPT 4 and Claude 3 Opus on the more complex PRECIS-2 tasks.
Pages: 1 2 3 | Single Page
No Responses to “Can AI Critically Appraise Medical Research?”