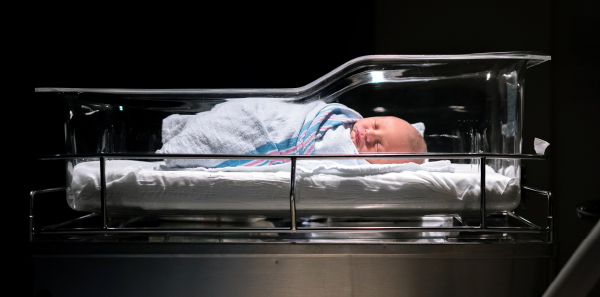
Machine Learning
All may not be lost, however. The high-quality prospective data collected by PECARN lends itself to reanalysis by what is rapidly becoming the new standard: machine learning (ML). In a subsequent analysis, informaticians applied three different ML techniques to the data from febrile infants: random forest, support vector machines, and neural networks.7 In their comparison, the authors found the random forest model to be best, able to produce a sensitivity of 98.6 percent while improving the specificity to 74.9 percent, exceeding the 30 to 60 percent specificity range of the original and other models. These models await further external validation.
Explore This Issue
ACEP Now: Vol 40 – No 03 – March 2021These data were not the only PECARN set to get the ML treatment this past year. Data from the PECARN’s blunt abdominal injury data set were evaluated using similar methods, producing advancements over the original analysis.7 Dueling analyses looking at the PECARN traumatic brain injury data found mixed results. An optimal classification tree showed potential improvements over the initial PECARN instrument in terms of specificity, while a second analysis of multiple ML methods came up mostly empty.8,9 Regardless of whether a bedside tool is ultimately created from these reanalyses, these alternate approaches to rich existing data add new insight and utility where previous efforts may have failed to show a path forward.
Equity
Lastly, and on an entirely different topic, PECARN has also been focusing on equitable care of children with acute injuries. A new publication looks at variability in opioid prescribing for children with long-bone fractures.10 Overall, 15 percent of 5,916 children seen in the emergency department for long-bone fractures were discharged with a prescription for opioid analgesia, with oxycodone the most frequently prescribed. Within the limitations of a retrospective analysis, independent factors associated with lower administration of opioid analgesia included non-white ethnicity and government health insurance. The appropriateness of opioid prescribing notwithstanding, there should be minimal variability across the socioeconomic spectrum, and variation should be driven by clinical features alone.
We are fortunate that the dedicated professionals of the PECARN group continue to advance emergency care for children and look forward to what new data they may bring to light in 2021.
The opinions expressed here are solely those of Dr. Radecki and do not necessarily reflect those of his employer or academic affiliates.
References
- Alpern ER, Kuppermann N, Blumberg S, et al. Time to positive blood and cerebrospinal fluid cultures in febrile infants ≤60 days of age. Hosp Pediatr. 2020;10:719-727.
- Florin TA, Ramilo O, Hoyle Jr JD, et al. Radiographic pneumonia in febrile infants 60 days and younger [published online ahead of print July 18, 2020]. Pediatr Emerg Care. doi:10.1097/PEC.0000000000002187.
- Florin TA, Ambroggio L, Lorenz D, et al. Development and internal validation of a prediction model to risk stratify children with suspected community-acquired pneumonia [published online ahead of print Nov. 7, 2020]. Clin Infect Dis. doi:10.1093/cid/ciaa1690.
- Kuppermann N, Dayan PS, Levine DA, et al. A clinical prediction rule to identify febrile infants 60 days and younger at low risk for serious bacterial infections. JAMA Pediatr. 2019;173(4):342-351.
- Velasco R, Gomez B, Benito J, et al. Accuracy of PECARN rule for predicting serious bacterial infection in infants with fever without a source. Arch Dis Child. 2021;106(2):143-148.
- Ramgopal S, Horvat CM, Yanamala N, et al. Machine learning to predict serious bacterial infections in young febrile infants. Pediatrics. 2020;146(3):e20194096.
- Pennell C, Polet C, Arthur LG, et al. Risk assessment for intra-abdominal injury following blunt trauma in children: derivation and validation of a machine learning model. J Trauma Acute Care Surg. 2020;89(1):153-159.
- Bertsimas D, Dunn J, Steele DW, et al. Comparison of machine learning optimal classification trees with the pediatric emergency care applied research network head trauma decision rules. JAMA Pediatr. 2019;173(7):648-656.
- Rowe C, Wiesendanger K, Polet C, et al. Derivation and validation of a simplified clinical prediction rule for identifying children at increased risk for clinically important traumatic brain injuries following minor blunt head trauma. J Pediatr. 2020;3:100026.
- Drendel AL, Brousseau DC, Casper TC, et al. Opioid prescription patterns at emergency department discharge for children with fractures. Pain Med. 2020;21:1947-1954.
Pages: 1 2 3 | Single Page
No Responses to “Insights from the Pediatric Emergency Care Applied Research Network”