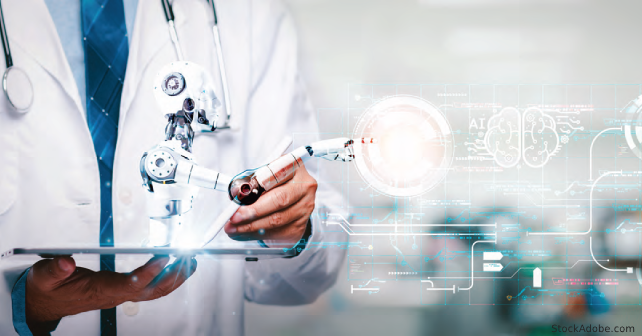
Virtually everyone has experimented with large language models (LLMs) in some fashion. Whether a person is conducting such parlor tricks as generating “Choose Your Own Adventure” children’s stories, looking for recipes in order to utilize a strange assortment of leftover ingredients, or composing eloquent poetry, the list of non-serious uses is vast. Putting the LLM to work, however, requires a substantial step up from these applications.
Explore This Issue
ACEP Now: Vol 43 – No 07 – July 2024Interest in LLMs for tasks beyond the chatbot realm stems from recognition that the underlying machine learning (ML) structure enables their use as generalizable prediction engines.1 Other, non-transformer-based ML methods can be used on clean, well-structured data sets, but LLMs are frequently capable of feats of unanticipated competence right out of the box. Owing to general availability, most of these studies evaluate the use of ChatGPT (created by OpenAI) as the testbed for processing text extracted from the electronic health record, although experiments from non-English-speaking countries utilize other LLMs.
Recently published experiments with LLMs in the emergency department fall into a handful of primary areas of interest. The first seems to be the use of LLMs as agents for triage and risk stratification. One of these studies evaluated the use of LLMs to return a Canadian Triage and Acuity Scale (CTAS) score.2 In it, authors developed six different prompts based on 61 previously validated clinical vignettes with corresponding CTAS scores. The goal of the varied prompt development was to determine to what extent the prompt format could either improve or diminish the accuracy and reproducibility of the results.
The experiment on CTAS scores was grossly unsuccessful, with an accuracy of less than 50 percent and repeatable variation of 20 percent. This sort of application illustrates where general models are limited by the nature of their basic design and shows that calculations are particularly challenging. In this case, the LLM lacks specific domain training on the CTAS score, and the predictions do not have the appropriate foundational training to operate accurately. These data do not indicate that an LLM cannot be a useful tool at triage, but that the use of general purpose LLMs will not be sufficient.
In a similar vein, another study evaluated the ability of an LLM to assess “clinical acuity” in the emergency department.3 Taking a different approach, this study did not utilize information available at triage, but instead used full-note text from completed documentation of history, examination, and assessment. Rather than being directly applicable to triage, then, this study aimed to test whether an LLM can grossly estimate clinical acuity in general, using the Emergency Severity Index as the “gold standard.”
Pages: 1 2 3 4 | Single Page
No Responses to “Tread Cautiously with Artificial Intelligence in Clinical Scenarios”