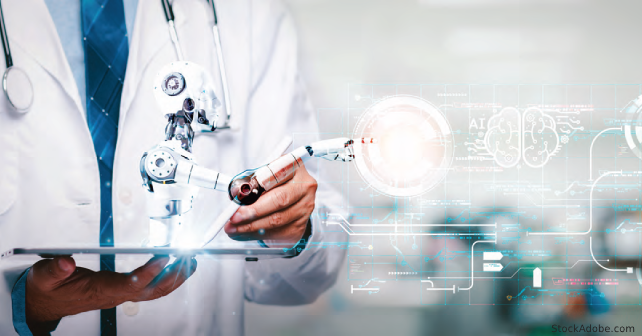
Here, the results tilted more favorably towards LLMs. Given pairs of patients with differing acuity as classified by ESI, the LLM was able to discern the patient of higher acuity approximately 90 percent of the time. This performance was roughly comparable with a corresponding physician reviewer in a subset of clinical notes undergoing manual review. These findings are less applicable to triage and demonstrated that identifying the “sicker” patient is still a leap away from clinical use. However, this nominal success represents the potential for future LLM deployment in this space.
Explore This Issue
ACEP Now: Vol 43 – No 07 – July 2024Last, with respect to triage, a rather interesting article from Korea described passive augmentation of the triage process.4 Using fine-tuned versions of Korean language-specific LLMs, this study demonstrated its use as a passive listener to medical conversations. Using text generated from listening to clinical interviews, including both human and automated text-to-speech transcriptions, these authors evaluated the ability of the LLM to identify the top three most clinically important utterances.
Following a fine-tuning process for these models, the authors were able to determine the best specific model, KLUE-RoBERTa, and found moderate similarity between human and LLM rankings of clinical importance. Interestingly, as a next step, both human reviewers and the LLM were prompted to provide an explanation regarding their selections of clinical importance. These explanations were used for a sort of Turing test, in which additional reviewers rated the quality of the explanations. Although humans provided more appropriate explanations, the differences were not particularly profound. As a mechanism for augmenting clinical operations, this approach may show value in passively collecting and feeding information into other systems.
The next area of attempted augmentation lay in summarization tools attempting to assist the over-burdened clinician. These include tools for synthesizing free-text data to assist in risk stratification, such as in a study evaluating the ability to automate HEART (history, ECG, age, risk factors, and troponin) score determination.5 In this study, the authors developed a framework within which to iteratively refine LLM prompts to automate determination of the HEART score. These prompts were tested on batches of synthetic clinical notes for four hypothetical patients. The goal of having several notes available was to test the ability of the LLM to combine multiple sources of data into its calculations.
Unlike the triage calculations, this demonstration was a bit more successful. Most prominently with GPT-4, the iterative prompt design process improved effectiveness, resulting in a mean error in calculating any individual subscore of only 0.10. Ultimately, in their test cases, each hypothetical patient was placed in the correct HEART score risk bin. Although this appears impressive, these results are not robust enough to generalize them to using GPT-4 in real-world applications, but, rather, they serve as a better example of the necessity of tuning the prompts used to digest clinical data for specific use cases.
Pages: 1 2 3 4 | Single Page
No Responses to “Tread Cautiously with Artificial Intelligence in Clinical Scenarios”