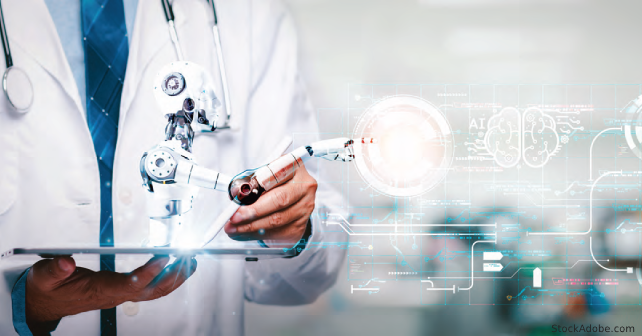
Another summarization tool is recently described in a preprint of the use of LLMs to create an ED discharge summary.6 Although many electronic medical records can generate generic discharge paperwork, a concise textual summary of a visit represents a time-consuming burden for clinicians. In this instance, GPT-3.5 and GPT-4 were used, and only one generic “write me a discharge summary” prompt preceded the text of the clinical note. Human reviewers rated each summary on accuracy, presence of AI hallucinations, and omissions of relevant information.
Explore This Issue
ACEP Now: Vol 43 – No 07 – July 2024In this simplistic experiment, the authors reported only 10 percent of 100 sample GPT-4 summaries contained inaccuracies, defined as reported facts incongruent with the original clinician note. These results represented the performance high point, however, with 42 percent of summaries exhibiting hallucinations and 47 percent containing clinically important omissions. The examples provided by the authors include all manner of misreported physical findings, confabulated follow-up recommendations, and elements mixed among sections of the clinical note.
As usual, this sample of published literature represents just a snapshot of work in the field. The evolving capabilities of LLMs far outpace the ability of researchers to test and report their performance. Most importantly, these articles demonstrate the need to cut through the hype and perform objective measurement prior to considering real-world use. Individual clinicians ought to use an abundance of caution when experimenting with publicly available LLMs in their own practice.
Dr. Radecki (@emlitofnote) i s an emergency physician and informatician with Christchurch Hospital in Christchurch, New Zealand. He is the Annals of Emergency Medicine podcast co-host and Journal Club editor.
References
- Jiang LY, Liu XC, Nejatian NP, et al. Health system–scale language models are all-purpose prediction engines. Nature. 2023;619(7969):357-362. doi:10.1038/s41586-023-06160-y.
- Franc JM, Cheng L, Hart A, et al. Repeatability, reproducibility, and diagnostic accuracy of a commercial large language model (ChatGPT) to perform emergency department triage using the Canadian triage and acuity scale. CJEM. 2024;26(1):40-46.
- Williams CYK, Zack T, Miao BY, et al. Use of a large language model to assess clinical acuity of adults in the emergency department. JAMA Netw Open. 2024;7(5):e248895.
- Lee S, Lee J, Park J, et al. Deep learning-based natural language processing for detecting medical symptoms and histories in emergency patient triage. Am J Emerg Med. 2024;77:29-38.
- Safranek CW, Huang T, Wright DS, et al. Automated HEART score determination via ChatGPT: Honing a framework for iterative prompt development. J Am Coll Emerg Physicians Open. 2024;5(2):e13133.
- Williams CYK, Bains J, Tang T, et al. Evaluating large language models for drafting emergency department discharge summaries. Health Informatics; 2024.
Pages: 1 2 3 4 | Single Page
No Responses to “Tread Cautiously with Artificial Intelligence in Clinical Scenarios”